The Path to AI-driven Drug Discovery - Part 1: What is Drug Discovery?
The road to a new drug molecule is long and winding. Currently, this long process is needed to tackle the diseases that plague mankind, such as cancer, neurodegenerative or cardiovascular diseases. In this 3-part series of Drug Discovery posts, we will be summarising the steps in the drug discovery pipeline and show how our technology at DeepMirror can de-risk and fast-track the process. In this first blogpost, we introduce the classical drug discovery pipeline as background and we provide the context for Part 2, where we will dive into how AI is revolutionising drug discovery. Part 3 will present a case study on how our technology can be used to accelerate drug discovery.
Drug discovery is the process by which a new molecule is identified and optimised to treat patients for a specific disease. Drug molecules can be small in size such as small molecules, or large macromolecules of biological nature such as antibodies, antibody-drug conjugates, RNA or proteins. Before the advent of modern drug discovery, finding new treatments relied on identifying active molecules from natural sources (such as plants) or on serendipity. For example, salicylic acid, the active molecule in aspirin, is found in willow tree bark and has been used for over 1500 years in different forms, until it was isolated in the 1820s, and modified by Friedrich Bayer and Company in the late 1800s to reduce gastrointestinal symptoms [1]. A well known example of a drug discovered by serendipity is penicillin, when in 1928 Alexander Fleming returned from holiday to find a type of mould growing on his plates which had inhibited bacterial growth. He extracted the molecule released by the mould, and it became the first antibiotic in the history of human medicine [2]. About 50% of new FDA-approved drugs from 1981 to 2010 were derived from natural products (also called semi-synthetic analogues) [3].
However, with advances in synthetic chemistry since 1970, the repertoire of synthesisable molecules has become increasingly complex and diverse. More molecular possibilities meant there was a requirement for more sophisticated large-scale screening. Luckily, from the late 1980s, the advent of in vitro screening, miniaturization and automated high-throughput techniques enabled testing of large amounts of well defined small molecules. These two improvements together meant many pharmaceutical companies were able to move away from natural product drug discovery and its inherent issues involving off-target effects, toxicology, DMPK, purification, and high synthesis costs.
With growing understanding of the biology underpinning disease mechanisms over the last 50 years, another foundational change occurred: instead of phenotypic screening (where the biological target is not known), pharmaceutical companies began to favour “target-based drug discovery” [3]. A target is a gene, or a gene product such as a protein, known to be involved in a disease. The ability to select a specific target meant drug screens could be planned more effectively. Still, target selection constitutes the riskiest step in drug discovery, where a considerable amount of time is invested. Selecting a wrong target leads in most of the cases to clinical failure.
The roadmap to small molecule drug discovery
Drug discovery is a highly capital-intensive market, with a new medicine requiring on average 10-15 years and more than 1bn€ before it reaches the shelves [4]. Worldwide revenues of pharmaceutical companies totalled 1.42 trillion U.S. dollars in 2021 [5]. Here we highlight the key steps in the drug discovery process and present an example of how this may look like in the real world (Fig. 1).
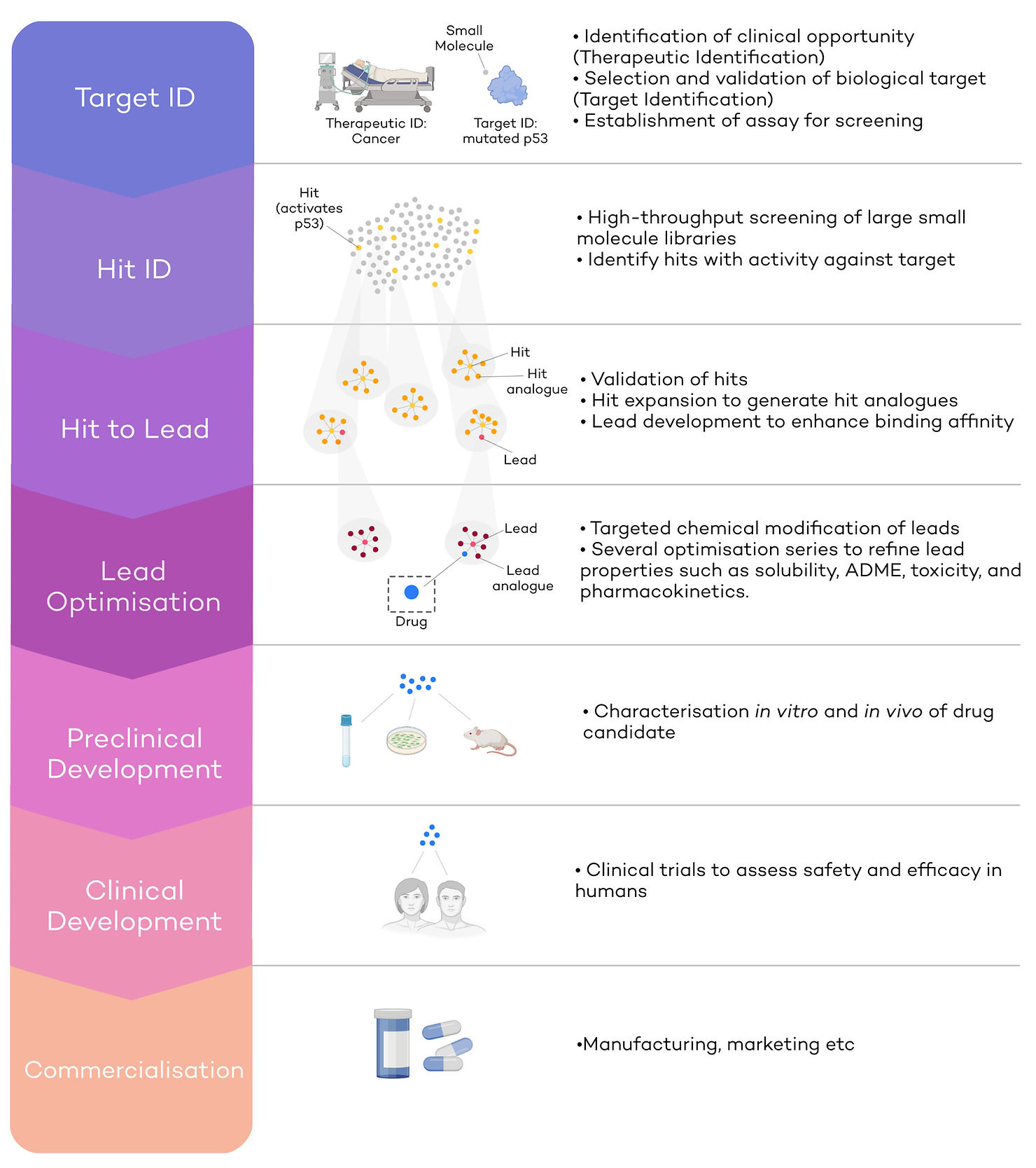
Therapeutic and Target identification (Target ID)
Cancer is one of the defining diseases of the modern world: 1 in 2 people will be diagnosed with cancer at some point in their lifetime [6]. Hence, the development of anti-cancer drugs makes up nearly 40% of the global drug discovery pipeline, and the number of anti-cancer compounds under development has quadrupled since 1996 [7]. Despite these leaps, cancer is still responsible for 1 in 4 deaths in the UK [8], and a pressing need for more and better therapies remains. Cancer would be the therapeutic ID, an opportunity where developing a new therapeutic would lead to the clinical improvement of a large population of patients. In other words, cancer would be the indication for which we are seeking to identify a target.
The next step is target identification. This step entails finding a biological target involved in cancer against which scientists can develop a drug. For example, p53 is a tumour suppressor protein considered the ‘guardian of the genome’ and is a promising, yet challenging, target for drug discovery, given no p53 inhibitors have been approved yet [9]. Healthy p53 protects cells from accumulation of mutations, by triggering cell cycle arrest or cell death (apoptosis). Mutations in TP53 (the gene that encodes p53) lead to p53 inactivation, which in turn causes an accumulation of DNA mutations within cells and unrestrained growth [9]. In this case, mutated p53 would be a biological target against which to initiate a drug discovery pipeline (Target ID).
At this stage, a robust assay to test the activity of a small molecule against p53 must be developed to be able to progress to screening stages. This happens before the hit identification phase; without robust assays which can identify hits, no progress in a drug discovery program can be made.
Hit Identification (Hit ID)
The second step in drug discovery is to identify molecular hits employing the aforementioned robust assays. Hits will be molecules which have a desired effect against the target (in our example mutated p53). Small molecules constitute the starting points for new refined series of compounds. To identify hits effectively, a collection of small molecules (or “library”) is screened against mutated p53 (Fig.1 – second panel). Libraries are usually very large, and the process of Hit ID can be accelerated in several ways. Firstly, library screening is a highly automated and high-throughput process, where hundreds of thousands of molecules are tested using robotic systems [10]. Secondly, DNA-encoded libraries (DELs) are being increasingly used in Hit ID [11]. DELs consist of small molecules which have been ‘bar-coded’ with a short DNA sequence. By making every molecule traceable, more molecules can be pooled in the same mix and tested together, making screening faster and expanding capacity to libraries of billions, or even trillions of compounds [12]. Finally, virtual screening can also be carried out, where small molecule libraries are tested virtually for predicted activity against the target.
Several ‘drug-likeness’ criteria are usually considered when designing libraries, which may include molecular mass, solubility, potency, toxicity, DMPK, Pka etc. For example, most small molecule drugs currently on the market are between 200-600Da in weight because they must be orally bioavailable or even pass the blood-brain-barrier (BBB) [13].
In our case, small molecules that can activate p53 will be considered hits and moved on to the next stage for further development (Fig.1 – yellow dots). Most promising molecules usually have analogues (molecules with similar structures) in the screen that are also active, meaning they belong to a series of compounds that already show structure-activity-relationships (SAR). This does not mean that singletons (compounds that have no other active family members in the screen) cannot be developed.
Hit to Lead (H2L)
The main aim of H2L is to improve binding affinity of selected hits or series of hits, to expand the molecular features of the series and to establish robust SAR. To do so, either so EC50, IC50, or both, against the target (mutated p53) are determined. EC50 and IC50 is the concentration at which half of the maximum activation (EC50) or inhibition (IC50) is achieved. Series of hit analogues are synthesised during ‘Hit expansion’, yielding small molecules with similar core structures to the hit (Fig.1 – yellow and orange clusters). This will lead to SARs and a good understanding of what changes to the molecular structure of the series can lead to better molecules and which don’t. The optimization of SARs is multi-parameterized and other in vitro and sometimes even in vivo assays will be consulted to optimize the molecular structure. For example, efficacy in cell culture, selectivity to p53, metabolic stability, low toxicity, high cell membrane permeability etc [14]. The key aim of H2L is to improve the binding affinity from often micromolar IC50/EC50 values at the hit stage, to nanomolar values at the lead stage by screening hit expansion series [14]. Leads with higher binding affinity and improved parameters will be moved on to the next stage, lead optimisation.
Lead Optimisation (LO)
Lead optimisation takes the most promising lead series and further refines their biochemical, biophysical and in vivo properties. During lead optimisation, leads are subjected to further targeted, sometimes small, but difficult, chemical modifications. SARs will be improved as much as possible to the desirable characteristics, while reducing undesirable ones (Fig.1 – orange and red clusters). The focus in this phase not just lies on efficacy but also on in vivo pharmacokinetic and pharmacodynamic (PK/PD) and toxicology profiling. For example, we might be interested in increasing affinity to p53 even further, increase metabolic half-life to ensure the drug is retained in the body long enough, increase selectivity to p53 to prevent binding to anti-targets. At the same time, we might want to reduce toxicity and any off-target effects. At the end of lead optimisation, very few compounds of one or two series will be selected to progress into preclinical development (Fig.1 – blue dot).
Preclinical development
The aim of preclinical development is to assess a drug’s safety and efficacy in animals and to predict a human dosage that is nontoxic and efficacious. To do so, in vitro assays and in vivo testing in cells and animals are carried out to ensure clinical testing can take place in humans.
During this stage, estimations of scale-up feasibility will be performed, including a chemistry manufacturing control (CMC). During preclinical development, a single novel clinical candidate is selected (from the optimised leads) for which a patent is secured (if it has not been done in H2L or LO phase). After approval of an IND (investigational new drug) application by the governmental body, clinical development might start.
Clinical development
During clinical development, a drug’s efficacy and safety are tested in humans. Clinical trials are divided into 3 stages. In phase I clinical trials, new drugs are tested on a small group of healthy volunteers to assess safety, evaluate recommended dosage, and identify any side effects. Sometimes, biomarkers that can indicate efficacy of treatment can be identified at this stage. In phase II clinical trials safety and efficacy in larger group of patients are tested. In Phase III, the largest group of patients (sometimes several thousands) is tested across regions and countries. The drug is compared to an existing treatment or a placebo to assess efficacy and safety.
At the end of this process, a New Drug Application (NDA) containing all the safety and efficacy information is submitted and must be granted approval before commercialisation. Sometimes, after approval, a Phase IV clinical trial is carried out, collecting long-term follow-up data.
From classical drug discovery to AI-driven drug discovery
In this first blogpost of the series, we have briefly summarised what is a decade-long billion-dollar process. Drug discovery is an immensely resource-intensive process, and a multi-step, multi-dimensional, hugely complex optimisation problem. Fortunately, AI has entered the field of drug discovery in the last few years with the promise of supercharging the identification and development of promising leads and unlocking difficult to treat targets.
At DeepMirror, we are working on democratising the field by making cutting edge graph semi-supervised machine learning algorithms easily useable by drug discovery scientists. This technique uses structural simulations to excel on small datasets and can thus be used from day 1 in the drug discovery pipeline (click here to learn more). In the upcoming posts, we will show where we fit into the drug discovery process (part 2) and how Small Data AI outperforms other state-of-the-arts machine learning algorithms (part 3).
Stay tuned!
References
1. https://en.wikipedia.org/wiki/History_of_aspirin
2.https://www.sciencemuseum.org.uk/objects-and-stories/how-was-penicillin-developed
3. https://drughunter.com/are-drugs-becoming-more-lipophilic-over-time/
4. JAMA, 2020: https://jamanetwork.com/journals/jama/issue/324/15
5. https://www.statista.com/statistics/263102/pharmaceutical-market-worldwide-revenue-since-2001/
6. https://www.nhs.uk/conditions/cancer/
8. https://www.cancerresearchuk.org/health-professional/cancer-statistics/mortality
9. Hassin, O. & Oren, M. Drugging p53 in cancer: one protein, many targets. Nature Reviews Drug Discovery 2022 1–18 (2022) doi:10.1038/s41573-022-00571-8.
10. https://en.wikipedia.org/wiki/High-throughput_screening
11.https://cen.acs.org/articles/95/i25/DNA-encoded-libraries-revolutionizing-drug.html
12. https://en.wikipedia.org/wiki/DNA-encoded_chemical_library